Time: August 2014 – August 2015
Team Size: 4 (first stage), 3 (second stage), advised by Dr. Jeff Hancock
Role: Team Leader, Principal Investigator
Methodology: Surveys, Qualitative Coding, Quantitative Analysis, Secondary Research
Product: Academic paper submitted to ACM CHI 2016 conference, PowerPoint presentation of the research project
**The publication of this research project is in progress (Journal of Technology Science)
Background & Ideation
This project was inspired by the story of Madison Holleran. Madison seemed like a happy college student from her Instagram account. But behind the scenes, this bright student was struggling with mental health. Inspired by this story, we directed our attention to how human behaviors manifest on photographic based social network.
Through intensive literature review, I quickly realized that there were very few existing literature on our research topic. As photo sharing had become increasingly popular on social networks such as Facebook, Twitter, Instagram, and others, this area proved to be a very interesting and meaningful opportunity for further investigation.
Research Question
We were specifically interested in how self-presentation goals guide deception in Instagram posts. Our first research question was to find out the prevalence of deceptive behaviors and how people judge their own images as deceptive. Our second research question was to find out what aspects of Instagram images users consider deceptive.
Data Collection Methodologies
For both Study 1 and Study 2, we defined a deceptive photo as “any photo you upload that is a deliberate attempt to mislead viewers. Any photo, or accompanying caption, that you think will cause a false belief in the person viewing the photo should be rated as deceptive.”
Study 1
In our study 1, we recruited participants from Amazon Mechanical Turk (MTurk) (N=213). Participants completed a survey that requires them to upload the nine most recent Instagram posts from their own account (see below). They were asked to rate the accuracy of each photo. If the photo was rated as inaccurate, participants were asked to explain the reasons why each photo was inaccurate.
I was able to understand the prevalence of deceptive behaviors by checking what percentage of photos were marked as deceptive. Also participants’ reasoning on their own photos gave me a clear picture of how they perceive their own photos.
Data collected from study 1 was analyzed by using descriptive and inferential statistics.
* Note: Instead of asking users how deceptive their photos were, we asked them the reverse, i.e. how accurate their photos were. From user interview and literature reviews, we found that people are reluctant to rate their photo as deceptive (social desirability bias), and that relying on the accuracy rating instead could reduce this bias.
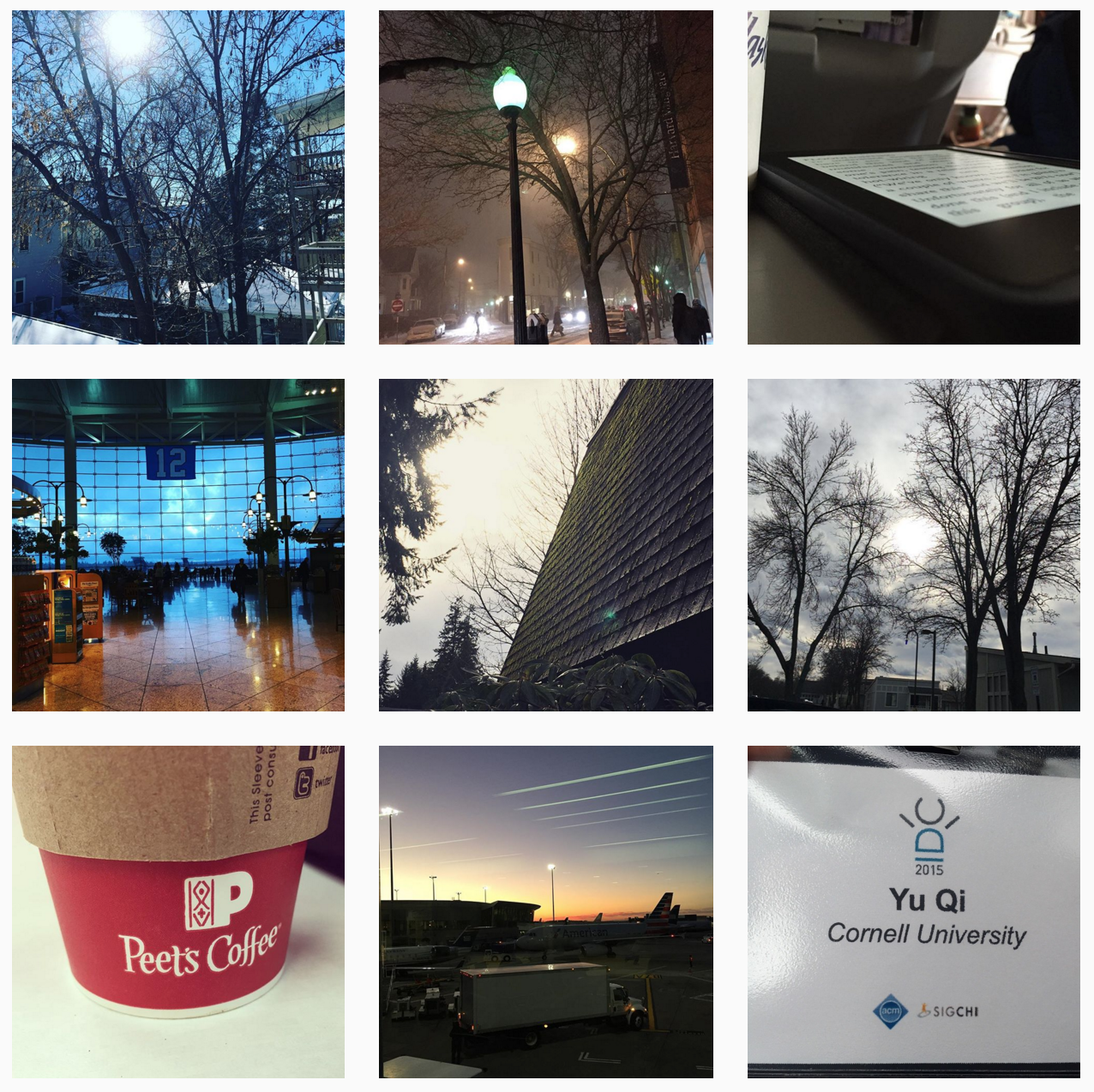
(From Yu's Instagram)
Study 2
The primary goal of study 2 was to examine in more detail 1) what aspects of their photo users judged as deceptive, and 2) what strategies people use to accomplish these deceptions.
Participants were recruited from a university campus (N=117). Instead of uploading the most recent nine instagram posts, participants uploaded four of their most deceptive Instagram posts and four of their most honest Instagram posts (in their own opinions).
We used a bottom-up approach to generate qualitative coding categories. There are two emerging patterns from our data, which are shown in the tables below.
Key Findings
From study 1, our data shows that Instagram users perceive filtering as deceptive, but as a less severe form compared to other forms of photographic deception.
From study 2, we found that captions were a surprisingly important strategy in deception on Instagram. While photographs on their own can only imply something misleading, users often accompanied a photo with an explicit lie. Despite the emphasis on photos on Instagram, the prevalence of deceptive captions (27%) suggests that textual components remain an important feature on Instagram.
Consistent with the results from Study 1, the use of filtering tools was the most common deceptive strategies.
Our data also shows the warrant effect that remarkably less deceptive photos tend to include people in the photo more often than honest photos. Similarly, another very interesting trend is that there are significantly more group photos in the honest photo category. Correlating this point to the trend of our data that honest photos tend to receive more likes, I can hypothesize that group pictures receive more likes. This is a potential direction for future researches.
Design Implications
Our result also surprisingly shows that the caption is an essential feature even in Instagram, where photography is supposed to be the focus. Users use captions to influence how other people perceive their pictures. My design recommendation is to make the caption a more prominent feature. For example, distinguish the format of the first comment from the caption, as the example shows below.
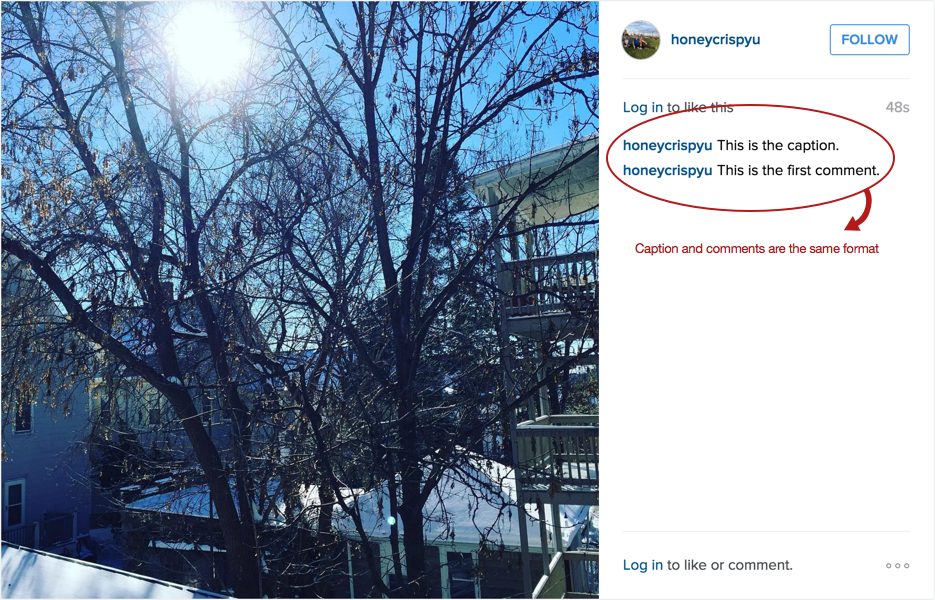
Based on our data that group photos are more likely to be perceived as honest, another design recommendation is that Instagram can help to promote honest behaviors by showing more group pictures in a user's newsfeed.
Considering together the finding that the use of filtering tools was the most common deceptive strategy and the tendency for honest photos to receive more likes, my last design recommendation is for Instagram to provide more filters that only slightly alter the photo.